AI’s Approach to Diversity and Inclusion
AI WORLDFEATURED
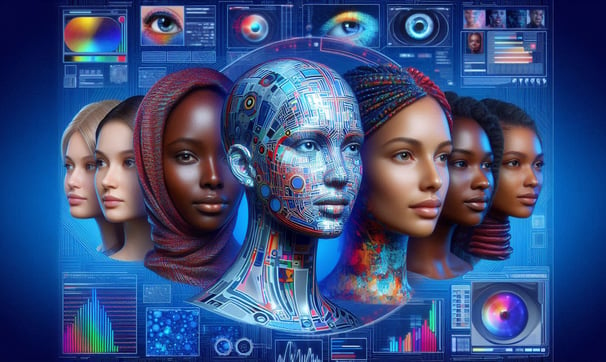
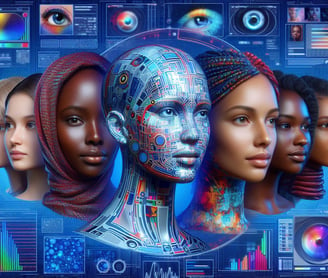
Artificial intelligence (AI) algorithms are increasingly However, as AI technologies become more embedded in daily life, questions regarding diversity and inclusivity in these systems have emerged.
Artificial intelligence (AI) algorithms are increasingly influential in shaping modern societies, from enhancing decision-making processes in healthcare to facilitating more efficient financial transactions. However, as AI technologies become more embedded in daily life, questions regarding diversity and inclusivity in these systems have emerged. The way AI algorithms handle diversity is crucial, as these systems impact hiring, healthcare, law enforcement, and social media—areas that inherently affect diverse populations. The tendency of AI, particularly in image recognition systems, to exhibit ethnic biases has led to scrutiny over whether AI is genuinely inclusive or merely perpetuating societal prejudices.
This article examines the role of AI in addressing diversity and inclusion, focusing on the pitfalls of image recognition technology, its failures in accurately identifying individuals across ethnic groups, and the subsequent need for deeper exploration and reform. By understanding the limitations and biases embedded within AI, we can create systems that better reflect and respect the multifaceted nature of human society.
Understanding Bias in AI and Its Origins
Bias in AI arises primarily from the data on which algorithms are trained. Most AI systems, including image recognition technologies, rely on extensive datasets to “learn” patterns and make predictions. However, if these datasets lack representation across diverse ethnicities, genders, or socioeconomic backgrounds, the resulting AI model is likely to reflect these imbalances. For instance, many widely used image recognition datasets contain a disproportionately high number of images from Western countries, leading to AI systems that perform accurately with lighter skin tones but falter when analyzing individuals with darker skin tones. This bias can produce adverse outcomes, reinforcing stereotypes or even endangering the individuals it misidentifies.
There are two primary sources of bias in AI systems. The first is data bias, which results from unbalanced datasets that lack representation from diverse demographic groups. The second source, algorithmic bias, arises when developers unintentionally or otherwise embed preferences into the algorithms. Algorithmic bias often reflects the values or assumptions of the people who create them, meaning that an overwhelmingly homogeneous development team may inadvertently create AI that fails to account for diversity.
Challenges in Image Recognition Systems
Image recognition systems, one of AI's most visible applications, have repeatedly faced scrutiny for their limitations in handling diversity. Research has shown that popular facial recognition systems have higher error rates when identifying people of color compared to their accuracy with lighter-skinned individuals. A 2018 study by MIT’s Media Lab, for example, found that certain facial analysis algorithms misclassified darker-skinned women nearly 35% of the time, while lighter-skinned men were identified with near-perfect accuracy. Such disparities can have serious consequences, particularly when these technologies are used in security settings, law enforcement, and public services.
These challenges stem from the fact that image recognition models are often trained on datasets that lack sufficient representation of all ethnic groups. This problem is exacerbated by the high computational costs associated with collecting and processing vast, diverse datasets. As a result, companies sometimes prioritize dataset size over diversity, inadvertently embedding biases that disadvantage underrepresented groups.
Consequences of Bias in AI
The consequences of biased AI systems are significant and extend beyond mere inaccuracies. When an image recognition system misidentifies a person of color, for example, it can lead to false arrests or wrongful accusations. In addition, the prevalence of bias in AI erodes trust in technology, particularly among marginalized communities who may already feel underrepresented or unfairly targeted. Moreover, biased AI systems in hiring or financial services can perpetuate historical inequalities, reinforcing systemic discrimination in ways that are difficult to detect and even harder to rectify.
In healthcare, biased AI systems may misdiagnose or overlook conditions that predominantly affect certain demographics, leading to poor health outcomes for these populations. For example, certain dermatology AI systems have struggled to diagnose conditions on darker skin tones accurately, leading to healthcare disparities that exacerbate existing inequities. Thus, the impact of AI bias spans multiple sectors and highlights the need for a more inclusive approach to AI development and deployment.
AI's Role in Promoting Diversity and Inclusion
Despite the challenges, AI holds potential as a tool for promoting diversity and inclusion. When AI models are carefully constructed with diverse data and ethical considerations, they can help organizations detect biases, facilitate inclusive hiring, and personalize services for diverse populations. For instance, AI-driven tools can help eliminate certain biases in recruitment by focusing on objective performance metrics rather than demographic information. Furthermore, natural language processing (NLP) technologies can analyze content to detect and remove biased or harmful language, promoting a more inclusive online environment.
Inclusive AI development requires a commitment to diversity at every stage of the AI lifecycle—from data collection to algorithm design to testing. An inclusive approach involves not only using balanced datasets but also incorporating diverse perspectives within development teams. By doing so, organizations can develop AI systems that are sensitive to the needs and experiences of different communities and minimize the risk of perpetuating harmful stereotypes.
Addressing Ethical Concerns in AI Development
The ethical implications of AI-driven diversity initiatives are profound. As AI becomes more adept at categorizing individuals based on characteristics such as ethnicity or gender, questions arise regarding the right to privacy and autonomy. Many people are uncomfortable with the idea of AI systems categorizing them based on ethnicity, given historical misuse of such categorizations. To address these concerns, AI developers must establish clear guidelines that respect individuals' rights while striving for inclusivity.
Furthermore, it is essential to establish accountability mechanisms that enable individuals to challenge or correct AI-driven decisions that may adversely affect them. For example, if an AI system denies someone a loan based on its analysis of demographic data, that individual should have the right to appeal the decision. Establishing these mechanisms requires a commitment to transparency, enabling users to understand how AI systems operate and how they reach decisions. Additionally, organizations should employ third-party audits to detect and correct biases in their AI models, ensuring ethical standards are consistently upheld.
Case Studies of AI Diversity Initiatives
Several tech companies have made strides in creating more inclusive AI systems. For instance, Google and IBM have both implemented initiatives aimed at reducing bias in their image recognition software. IBM has worked on developing a dataset called “Diversity in Faces,” which includes images of individuals from a broad spectrum of ethnic backgrounds. This dataset aims to improve the accuracy of facial recognition systems across different demographics.
Similarly, Microsoft has updated its facial recognition technology to improve accuracy for darker-skinned individuals, after facing criticism for its initial biases. The company has also adopted an “AI Ethics Committee” that reviews its AI projects to ensure they meet ethical standards, particularly regarding diversity and inclusion. These initiatives demonstrate that addressing bias in AI is not only feasible but can also serve as a competitive advantage for companies committed to ethical AI development.
The Path Forward: Recommendations for Inclusive AI
To advance diversity and inclusion in AI, developers and organizations must take several proactive steps:
Prioritize Representative Data Collection: Companies should invest in creating and utilizing datasets that reflect the diversity of the populations they serve. This approach ensures that AI models are trained on data that accurately represents all demographic groups.
Establish Inclusive Development Teams: Diversity within AI development teams is crucial. By incorporating diverse perspectives, teams can identify and address biases that may go unnoticed in homogeneous groups.
Implement Fairness Metrics: Organizations should adopt fairness metrics to evaluate the performance of their AI models across different demographic groups. These metrics help identify areas where the model may exhibit biases, allowing for adjustments to improve inclusivity.
Conduct Regular Audits: Third-party audits can provide unbiased assessments of an AI system’s fairness and inclusivity. Regular audits ensure that biases are detected and addressed throughout the AI’s lifecycle.
Enhance Transparency and Accountability: Organizations should be transparent about how their AI systems work, including the data sources and algorithms used. Clear documentation and explanation help build public trust in AI technologies and allow users to understand and challenge AI-driven decisions.
Conclusion
As AI continues to shape society, the importance of diversity and inclusion in these technologies cannot be overstated. While current AI systems face significant challenges in accurately representing diverse populations, the technology’s potential to foster inclusivity is undeniable. Through representative data collection, inclusive development practices, and ongoing ethical considerations, we can build AI systems that not only recognize the richness of human diversity but also support and celebrate it. Ensuring that AI systems treat all individuals fairly and respectfully is not merely an ethical obligation—it is a fundamental requirement for creating technologies that serve society as a whole.
By addressing these challenges head-on, the AI community can build systems that genuinely respect diversity and inclusion, paving the way for a future where AI empowers rather than marginalizes.